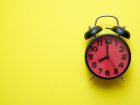
By Gladimyr Sully, Financial Data & Analysis, idaciti; Christine Tan, Co-founder and Chief Research Officer, idaciti; and Elaine Zhang, Financial Data Analyst, idaciti
What if you could update your earnings model within 60 seconds of the SEC publishing the company’s 8-K to the SEC’s EDGAR system? What if you could do that for every company in your portfolio automatically, as each company reports?
It can be done. On March 11, 2021, Tilly’s, Inc., a small-cap, specialty retailer, submitted their Earnings Form 8-K to EDGAR, and it was accepted by the SEC at 16:13:34 (4:13 PM ET) as shown in the EDGAR System screen shot below.
By 4:14 PM ET, the operating results data were extracted, the earnings model updated, and trend analysis charts revised to add the new data-points. Analysis finalized and decisions made by 4:15 PM!
Although earnings announcements are not published in a standardized, machine-readable format, XBRL provides a framework that makes this feasible. And as you can see from the example shown, this kind of automated data extraction can be performed for retail companies as small as Tilly’s or as big as COSTCO or BJ’s Wholesale.
The value of machine-readable earnings announcements
Securities analysts are in a race with the clock during earnings seasons. Although data in public company 10-Ks and 10-Qs is in fully machine-readable, structured XBRL format, earnings announcements (either in Form 8-K or newswire versions) are not. They are more timely, but they are available only as text or HTML files which are typically downloaded and manually rekeyed into earnings models.
idaciti is a financial data and software solution provider that utilizes XBRL to eliminate scraping filings' via a manual process. Our clients want timely, accurate, and transparent access to data embedded in the earnings announcements. Because our business is leveraging XBRL-formatted data, we know that the consistent structure of XBRL provides a perfect framework to structure unstructured data.
The XBRL framework
The XBRL specification has a consistent method to embed metadata into each value reported. For example, the value “7485” for Receivables, shown below in Tilly’s Balance Sheet on the Commission's website through their SEC Viewer, can be understood by a computer (even though the computer can’t read the rows and column headers on the table). That's because XBRL requires properties of the value to be reported along with the value itself.
Tilly’s 10-Q was prepared using Inline XBRL, which lets visitors see the human-readable financial statement table, and also "check under the hood" into the metadata reported along with that value. The gray popup box in the SEC Viewer shown below allows us to see that the value "7485” represents Receivables, for the period ending February 1, 2020, reported in thousands of US dollars; and it is a debit balance. Transporting these characteristics of the value allows it to be unambiguously machine-readable.
Ideally, the earnings release would be prepared by issuers in XBRL format, just like the 10-Ks and 10-Qs they submit today. That would improve the data's usability and ensure that the issuer's message is conveyed accurately and clearly.
But given our experience with XBRL, we leveraged the XBRL framework to do the next best thing.
Structuring unstructured data
We identify the facts reported in the earnings announcements, compare them against previously submitted 10-Qs or 10-Ks (as shown in the figure below), and use a combination of machine learning techniques; the rich metadata of existing US-GAAP XBRL tags; and our created algorithms to auto-tag the earnings releases.
The data is transformed from unstructured to structured, driving the earning model. It can now be measured and analyzed like the XBRL formatted 10-K or 10-Q reported values.
Handling Non-GAAP Financial Measures
Earnings releases often contain some non-GAAP financial measures (NGFM), which do not have associated XBRL tags. An example of this can be seen in Adjusted EBITDA on the earnings release for BJ's WHOLESALE CLUB HOLDINGS below.
NGFM are valuable metrics to investors; they provide additional insight into the performance of company results. The XBRL framework's extensibility allows for creating new company-specific XBRL concepts to collect these critical values.
We utilize our combination of machine learning and created algorithms to assign XBRL metadata to these reported values. The result is that unstructured NGFM data become structured with the same level of interactivity as the US-GAAP XBRL values.
Shouldn’t Issuers XBRL-Format their Own Earnings Release?
Absolutely. Ideally, issuers would prepare their earnings announcements in XBRL format, just like they do with the periodic filings. That would ensure the highest level of speed and integrity to the data, and greatly enhance the earnings release value.
But in the meantime, XBRL can still help. It gives us a framework to report data, even unstructured data, in a structured format. Creating machine-readable data out of unstructured earnings releases takes us about 60 seconds after the data becomes available from the SEC EDGAR System and generates a file like the one below on the idaciti platform.
As soon as the earning release is published on EDGAR, our system grabs it and runs the XBRL framework against it to generate tagged data and extracts that data into different types of analyses, like the time series of net inventory found on the bottom left.
Investors and researchers need the accuracy and speed that only machine-readable data can provide. Our clients have the unique ability to begin performing analysis immediately with our structured data-first approach.
It’s not magic. It’s XBRL.
This is the first in a multi-blog series on earnings announcements and XBRL. Watch for the next post in the coming weeks.